How mathematical modelling shapes public health policies: cervical cancer prevention
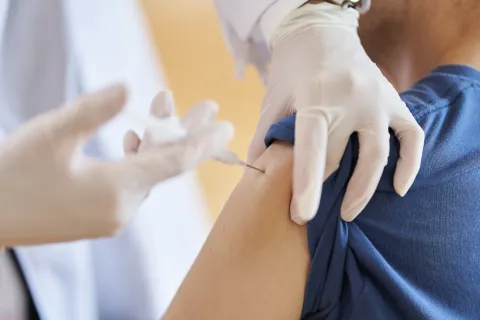
Mathematical modelling is used to inform decision making in public health by formalising assumptions, simulating the impact of interventions such as screening and vaccination, and suggesting what might happen in the future under different interventions. This has been crucial in expanding HPV vaccination to prevent cervical cancer.
Mathematical models have shaped responses to disease outbreaks such as COVID-19, and have also guided cancer prevention programmes such cervical screening and HPV vaccination, highlighting their critical role in health strategy development
Mathematical models are valuable tools in shaping public health policies. From predicting the spread of infectious diseases to evaluating the cost-effectiveness of health interventions, these models provide critical insights that guide decision-making processes.
During the COVID-19 pandemic, mathematical models were cast into the spotlight. They became instrumental in informing lockdown strategies, vaccination rollouts, and resource allocation in many settings.
However mathematical modelling has been used to inform decision making in public health long before this and continues to play a critical role in informing policy in many other areas today.
Mathematical modelling allows us to formalise our assumptions, simulate the impact of interventions like screening and vaccination, and then simulate forward in time to suggest what might happen in the future under different interventions.
In my own area as a researcher in cervical cancer prevention, mathematical modelling has been used to inform governments of optimal cervical cancer prevention strategies, providing estimates of costs, lives that would be saved, and harms associated with a range of interventions under consideration.
It is often the case that policy makers and advocates will require evidence to support health prevention investment specific to their setting, including evidence that a particular intervention is cost-effective and feasible for the setting, and this is one approach in which modelling can be useful.
In 2014, my colleagues and I worked closely with Australia’s Medical Services Advisory Committee, using modelling to simulate over 100 cervical screening interventions spanning different screening test technologies, screening ages and screening intervals. [Lew/Simms LancetPH]
The model, called Policy1-Cervix, was able to predict the rates of cervical cancer incidence and mortality, cervical precancer treatment rates, and costs associated with each of the strategies.
The modelling exercise concluded that primary HPV testing approaches were the most effective and cost-effective in both unvaccinated and vaccinated cohorts in Australia. These results underpinned the decision to transition from cytology screening to primary HPV screening in Australia’s National Cervical Screening Program.
More recently, the World Health Organization updated their cervical screening guidelines, and modelling, again, played a key role in informing this update.
In this exercise, using the Policy1-Cervix platform, we found that primary HPV testing approaches were more effective than primary VIA or cytology, minimised the harms associated with treatment for precancer, and were the most cost-effective across 78 low-and lower-middle income countries. [Simms/Keane 2023, Nature Medicine]
These predictions informed WHO’s updated cervical screening guidelines, launched in 2021, which now recommend using HPV as the primary screening test rather than VIA or cytology screening.
In addition to directly informing policy for governments and international organisation, modelling can be used to help understand the potential impact of aspirational targets, such as WHO’s global elimination targets for cervical cancer.
The Cervical Cancer Elimination Modelling Consortium, consisting of three independent modelling groups: The Daffodil Centre (Australia), University Laval (Canada) and Harvard (USA), simulated the impacts of implementing WHO’s elimination targets. [Canfell Lancet2020, Brisson Lancet2020]
The models predict that 72 million cases and 63 million deaths could be prevented over the next century if WHO’s elimination targets are met across all 78 low-and lower-middle income countries. These insights underpinned the WHO global strategy for elimination and provide quantitative insight into the importance of these interventions at a global scale.
Mathematical modelling can also have an impact on public perception. HPV vaccine hesitancy in Japan began in 2013 when reports of adverse events, such as chronic pain and movement disorders, emerged following vaccination. These reports, although not conclusively linked to the vaccine, received extensive media coverage and led to public concern.
Consequently, the Japanese government suspended its proactive recommendation of the HPV vaccine, causing a dramatic decline in vaccination rates – from more-than 70% coverage to less than 1% [Hanley Lancet 2015].
This hesitancy persisted despite subsequent studies reaffirming the vaccine's safety and effectiveness, posing significant public health challenges in preventing cervical cancer.
In 2019, we modelled the impact of seven years of missed vaccination from 2013-2019, and predicted that, over the lifetime of cohorts who missed vaccination, 5,000 lives would be lost. We also showed that 3,000 of these lives could still be saved if vaccination was rapidly scaled-up in 2020, including catch-up vaccination for missed cohorts.
This modelling gained substantial attention in Japanese social media and news media space.
Modelling quantified the impact of the hesitancy crisis, showing that 5,000 young girls would eventually die from the disease who otherwise would not have, had the crisis had been prevented. This helped to shine a spotlight on the impact of inaction and highlight the urgency of a reversal of the situation.
Since then, the government has re-instated active recommendations for the HPV vaccine; however, evidence of substantial changes in coverage are still forthcoming.
Mathematical modelling requires strong collaboration with experts across many disciplines. This includes working with epidemiologists who have strong knowledge of the evidence base to inform parameter estimates, and policy makers who can provide relevant context and highlight the important questions that are being grappled with.
It also requires clear communication of the modelling results, including limitations and key assumptions made throughout the process. [See HPV World.]
Finally, mathematical modelling provides stakeholders with a more concrete vision of the impact of their choices, both short and long term, leading to more informed decisions and better health outcomes.
Last update
Wednesday 10 July 2024Share this page